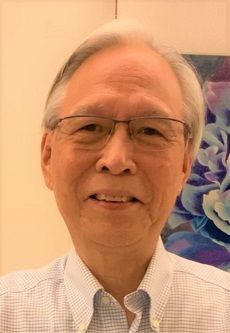
Xi-Ren Cao
The Hong Kong University of Science and Technology
Title: Optimization of Average-Cost Nonhomogeneous Markov Chains
Bio:
Xiren Cao obtained a PhD degree from Harvard University in 1984. He is now a Professor Emeritus of the Hong Kong University of Science and Technology. He was a consulting engineer for Digital Equipment Corporation, U.S.A, a research fellow at Harvard University, and a reader, professor, and chair professor at the Hong Kong University of Science and Technology. He owns three patents in data- and tele- communications and has published five books in the areas of performance optimization, discrete event dynamic systems, and stochastic learning and stochastic control. He is Fellow of IEEE and IFAC. His current research areas include stochastic control, financial engineering, stochastic learning and optimization, and discrete event dynamic systems.
Abstract:
Many real-world systems are time-dependent. In time-nonhomogeneous Markov chains (TNHMCs), the state spaces, transition probabilities, and reward functions depend on time. Notions such as stationarity, ergodicity, periodicity, connectivity, recurrent and transient states, which are crucial to the analysis of time-homogeneous Markov chains (THMCs), no longer apply. In addition, the long-run average criterion suffers from the so-called under-selectivity, which roughly means that it does not depend on the rewards received in any finite period. Dynamic programming may not be very suitable to address such optimization problems, and new notions and a different optimization approach are needed. In this talk, we show that confluencity captures the essentials of analysis of such systems. Confluencity refers to the property that two independent sample paths of a TNHMC starting from two different initial states will eventually meet together. With confluencity, the states in a TNHMC can be classified into different classes of confluent states and branching states, and the optimization conditions for the long-run average, bias, Nth-bias, and Blackwell optimality for single class and multi-class TNHMCs can be derived. In the analysis, the relative optimization approach is applied; the approach simply compares the performance measures of a system under any two policies. The under-selectivity is reflected in the conditions. The optimization of time-homogeneous systems becomes a special case.
Christos G. Cassandras
Division of Systems Engineering,
Department of Electrical and Computer Engineering, and
Center for Information and Systems Engineering (CISE)
Boston University
Brookline, MA 02446
[email protected], https://christosgcassandras.org
Title: Bridging The Gap Between Optimal And Real-Time Safe Control: Making Autonomous Vehicles A Reality
Bio:
Christos G. Cassandras is Distinguished Professor of Engineering at Boston University. He is Head of the Division of Systems Engineering, Professor of Electrical and Computer Engineering, and co-founder of Boston University’s Center for Information and Systems Engineering (CISE). He received a B.S. degree from Yale University, M.S.E.E from Stanford University, and S.M. and Ph.D. degrees from Harvard University. In 1982-84 he was with ITP Boston, Inc. where he worked on the design of automated manufacturing systems. In 1984-1996 he was a faculty member at the Department of Electrical and Computer Engineering, University of Massachusetts/Amherst. He specializes in the areas of discrete event and hybrid systems, cooperative control, stochastic optimization, and computer simulation, with applications to computer and sensor networks, manufacturing systems, and transportation systems. He has published over 450 refereed papers in these areas, and six books. He has guest-edited several technical journal issues and serves on several journal Editorial Boards. In addition to his academic activities, he has worked extensively with industrial organizations on various systems integration projects and the development of decision-support software. He has most recently collaborated with MathWorks, Inc. in the development of the discrete event and hybrid system simulator SimEvents.
Dr. Cassandras was Editor-in-Chief of the IEEE Transactions on Automatic Control from 1998 through 2009 and has also served as Editor for Technical Notes and Correspondence and Associate Editor. He is currently an Editor of Automatica. He was the 2012 President of the IEEE Control Systems Society (CSS). He has also served as Vice President for Publications and on the Board of Governors of the CSS, as well as on several IEEE committees, and has chaired several conferences. He has been a plenary/keynote speaker at numerous international conferences, including the American Control Conference in 2001, the IEEE Conference on Decision and Control in 2002 and 2016, and the 20th IFAC World Congress in 2017 and has also been an IEEE Distinguished Lecturer.
He is the recipient of several awards, including the 2011 IEEE Control Systems Technology Award, the Distinguished Member Award of the IEEE Control Systems Society (2006), the 1999 Harold Chestnut Prize (IFAC Best Control Engineering Textbook) for Discrete Event Systems: Modeling and Performance Analysis, a 2011 prize and a 2014 prize for the IBM/IEEE Smarter Planet Challenge competition (for a “Smart Parking” system and for the analytical engine of the Street Bump system respectively), the 2014 Engineering Distinguished Scholar Award at Boston University, several honorary professorships, a 1991 Lilly Fellowship and a 2012 Kern Fellowship. He is a member of Phi Beta Kappa and Tau Beta Pi. He is also a Fellow of the IEEE and a Fellow of the IFAC and holds a Chair Professorship at the Department of Automation, Tsinghua University.
Abstract:
Implementing solutions to complex dynamic optimization problems is limited by the fact that these solutions must often satisfy hard safety constraints at all times. A prime example arises in optimizing the operation of autonomous vehicles which must, above all else, guarantee safety. Obtaining such solutions incurs a high computational cost, which limits them to models with simple linear dynamics, simple objective functions, and ignoring noise. Control Barrier Functions (CBFs) may be used for safety-critical control at the expense of sub-optimal performance. We present a real-time control framework that combines trajectories generated through optimal control with the computationally efficient CBF method providing safety guarantees. A tractable optimal solution is first obtained for a linear or linearized system with few or no constraints. Next, this solution is optimally tracked while using CBFs to guarantee the satisfaction of all state and control constraints. This Optimal Control and CBF (OCBF) framework is applied to autonomous vehicles in transportation systems where the objective is to jointly minimize the travel time and energy consumption for each vehicle subject to speed, acceleration, and speed-dependent safety constraints. When considering complex objective functions, nonlinear dynamics, noise, and passenger comfort requirements, analytical optimal control solutions are simply unavailable. It is, however, possible, to adapt the OCBF approach to such problems. Simulation examples confirm that the resulting behaviors are not only provably safe but also perform significantly better than human-driven vehicles.
Eitan Altman
Title: Optimal Control and Game theory applied to Epidemics
Bio:
Eitan Altman received the B.Sc. degree in electrical engineering, the B.A. degree in physics, and the Ph.D. degree in electrical engineering from the Technion–Israel Institute of Technology, Haifa, 1984, 1984, and 1990, respectively, and the B.Mus. degree in music composition from Tel-Aviv University, Israel, in 1990. Since 1990, he has been a Researcher with the National Institute for Research in Computer Science and Control (INRIA). His articles (245 in international scientific journals and 423 in international conferences with peer reviews) have had a large impact and have in google scholar more than 21300 citations. He is the coauthor of four monographs which includes his book on the foundations of constrained Markov Decision Processes. His areas of interests include network engineering games, social networks and their control, and the analysis through game theoretical models of network neutrality issues. He received the Best Paper Award from Networking 2006, Globecom 2007, IFIP Wireless Days 2009, and CNSM 2011 (Paris) conferences. He has also received the Grand Prix de France Telecom from the French Academy of Sciences, in 2012, the ISAACS Award from the Society of Dynamic Games for his contribution in game theory, in July 2014, and the Distinguished Technical Achievement Recognition Award from the IEEE TC on Big Data conference (TCBD), for his outstanding technical leadership and achievement in stochastic modeling and big data analysis, in December 2017. He has been in the editorial boards of the journals Wireless Networks (WINET), Computer Networks (COMNET), Computer Communications (Comcom), Journal of Discrete Event Dynamic Systems (JDEDS), SIAM Journal of Control and Optimization (SICON), Stochastic Models, and Journal of Economic Dynamics and Control (JEDC). He is Fellow of IEEE. His detailed information can be found at http://www-sop.inria.fr/members/Eitan.Altman/
Abstract:
We shall present in this talk several epidemic models for developing insight on measures to defend against the population of viruses. In particular, we shall use the maximum principle of Pontryagin and HJB equations to derive the structure of the defence policies against the virus. In particular, we shall study asymptotic behaviour of epidemic systems with multiple communities obtained by slowing down the mobility between different communities. This is done by applying singular perturbation optimal control. We shall describe applications to both security in computer networks as well as to the fight against COVID-19.